Predictive Analytics
A prerequisite for the use of machine learning and algorithms is a well-maintained data warehouse in which data is brought together and managed. In more in-depth analyses, internal company data can additionally be expanded with external data about the location (geodata) or demographic aspects.
Based on this historical data, statistical models and machine learning techniques can be applied to make predictions about future events. Patterns can be identified in individual time series as well as in large customer data sets.
Regression in price management
A core technology of machine learning is regression. It learns from training data how a continuous value can be predicted. In pricing, these are usually optimal prices and discounts. This method is therefore ideal for supporting pricing in sales without explicit rule systems.
With peer pricing, machine learning can be used to generate price or discount suggestions at a “usual” level from available data. Machine learning can also be used to find optimal settings for target pricing systems and spare parts pricing logics.
Econometric models
Econometric models are used, for example, to forecast inventories or to estimate demand and commodity price developments.
These models are suitable for identifying patterns such as seasonalities or trends – so that, among other things, fluctuations in demand can be better anticipated.
Decision trees and clustering algorithms
Other methods – such as decision trees and clustering algorithms – are helpful in customer segmentation. Here, comparative values of customers with similar characteristics are used to make statements about existing and new customers with similar characteristics.
Improving the customer journey thanks to AI
Predictive analytics is about automatically evaluating events and calculating the most likely scenarios – the primary goal is to achieve the highest possible forecasting quality. These technical-looking processes can practically improve the daily customer journey.
Personalization of the customer experience
The targeted use of these methods leads to a significantly more individualized customer approach. For example, based on granular customer classification, each cluster can receive personalized mails and newsletters. If enough individual data is available, customers can even receive specific next-best-offer suggestions and discounts. This not only works for Netflix or Amazon but can also be applied in the marketing and sales of medium-sized companies – as long as past values about purchasing behavior have been systematically recorded. Suggestions of consumption bundles can be made both in the online store and in stationary retail by trained sales staff.
Possible applications of AI with new and existing customers.
Artificial intelligence and machine learning with the core technologies of classification, clustering and dimension reduction are applied to both new and existing customers.
Acquiring new customers / Big deal management
- The existing customer base can be used to generate probabilities of success for cold calling
- Especially for big deal price management, a high or low award probability is valuable information
- Offers can be prioritized based on the highest chance of closing (lead scoring)
- The most promising sales channels and means of communication can be identified on a customer-specific basis (opportunity scoring)
Build up existing customers
- Targeted customer classification and grouping is possible: R&P has, for example, developed a completely new AI approach for identifying price-sensitive spare parts commodity groups
- Based on the classification, the consistency of the discount and margin systems can be checked and adjusted if necessary
- The buying behavior of the individual clusters can be the basis for new product suggestions as well as cross- and up-selling activities (recommender system)
- The formation of product bundles (bundling) by association rules can also be supported in a target-oriented manner by a combination of BI tools and machine learning – in this way, correlations between different product options can be recognized and patterns in ordering behavior identified
- Personalized newsletters and online catalogs can be created based on affinity rankings per product
- The repurchase frequency through more targeted customer contact can be increased
Outlier detection: Detect outliers at an early stage
Algorithms can also help to uncover existing inconsistencies in price logics. Outlier detection is used to identify outliers and make existing grievances visible – unusual behavior patterns can be analyzed in greater depth in a next step. This is significantly more objective and time-saving than a spot check by a human.
Retain existing customers - Churn Prediction
Customer churn is a problem that every industry has to deal with. Particularly in times of declining customer retention and loyalty, preventing churn and cancellations is an essential component of a sustainable business strategy.
A churn prediction model provides information on the likelihood of customers switching to another provider in a given period. Both purchase history and data such as service visits and complaints provide information about current customer satisfaction. Historical customer churn serves as the basis for model calibration. The goal is clearly defined: The churn rate is to be continuously reduced (churn prevention).
Customer relationship management and churn prevention
Churn prevention only works if there is close integration with intelligent customer relationship management, in which clear instructions for action are defined. It is not worth stopping switching projects for all customers – the loss of a premium customer hurts more than the departure of a C-customer. The combination of customer segmentation and churn prediction model is the key to success. This information is particularly important for providers of contracts and subscriptions, such as energy suppliers, streaming services, banks and insurance companies, as well as in the software-as-a-service (SaaS) sector.
Identify terminations at an early stage
These models are to be understood as supporting tools so that sales staff and call center employees know which customers have a high churn risk. Once a customer has cancelled, it is usually difficult and costly to win them back. It is therefore a matter of implementing preventive actions to retain customers, so that cancellations are prevented even before customers themselves think about it. In online retailing, for example, individual discounts can be given automatically. The abstract probabilities can be translated into an easy-to-understand traffic light, which can serve as an early indicator. In the yellow and red categories, actions for customer retention can be carried out in stages.
Experience in the field of artificial intelligence
The decisive criterion for the success of these projects is transparency. If users can understand the logic of the algorithm, acceptance increases – a “black box” solution is usually doomed to failure.
Roll & Pastuch is the leading consultancy for quantitative methods in price management, sales and strategy. We offer pragmatic machine learning and AI solutions so that processes can be optimized and automated.
This ranges from quick checks (outlier detection, data audit) to extensive projects in the area of customer segmentation and churn prediction.
Use artificial intelligence in pricing and sales
We will be happy to answer your questions and provide you with further information.
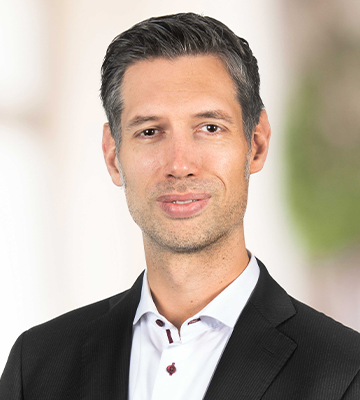
Kai Pastuch
Kai Pastuch is Managing Director of Prof. Roll & Pastuch. Before joining as Managing Partner, he was Director at a leading international strategy and marketing consultancy. As a graduate in business informatics, he also manages our software company nueprice, which specializes in the pricing of spare parts with the product of the same name. Mr. Pastuch has extensive project management experience from numerous projects for large international companies and German medium-sized businesses in the areas of price management, marketing, sales and strategy. In addition to numerous publications in renowned journals and the publication of the reference books Praxishandbuch Preismanagement and Big Deal Management, he is a sought-after moderator and speaker on all aspects of sales and pricing. As a practice-oriented manager, he likes to get personally involved in our projects and contributes his broad experience in workshops and steerings.